はじめに
前回は yolov5-tracking のデモを動かす方法について説明しました。
今回は、引数なしでコードを実行できるように変更していきます。
前提条件
前提条件は以下の通りです。
- Windows11
- Python3.9
- PyTorch == 1.12.1+cu113
プログラムの変更
早速、プログラムを変更していきます。
track.py をコピーして track_custom.py という名前に変更してください。
# limit the number of cpus used by high performance libraries
import os
os.environ["OMP_NUM_THREADS"] = "1"
os.environ["OPENBLAS_NUM_THREADS"] = "1"
os.environ["MKL_NUM_THREADS"] = "1"
os.environ["VECLIB_MAXIMUM_THREADS"] = "1"
os.environ["NUMEXPR_NUM_THREADS"] = "1"
import sys
sys.path.insert(0, './yolov5')
import argparse
import os
import platform
import shutil
import time
from pathlib import Path
import numpy as np
import cv2
import torch
import torch.backends.cudnn as cudnn
from yolov5.models.experimental import attempt_load
from yolov5.utils.downloads import attempt_download
from yolov5.models.common import DetectMultiBackend
from yolov5.utils.datasets import LoadImages, LoadStreams, VID_FORMATS
from yolov5.utils.general import (LOGGER, check_img_size, non_max_suppression, scale_boxes, #scale_coords,
check_imshow, xyxy2xywh, increment_path, strip_optimizer, colorstr)
from yolov5.utils.torch_utils import select_device, time_sync
from yolov5.utils.plots import Annotator, colors, save_one_box
from deep_sort.utils.parser import get_config
from deep_sort.deep_sort import DeepSort
FILE = Path(__file__).resolve()
ROOT = FILE.parents[0] # yolov5 deepsort root directory
if str(ROOT) not in sys.path:
sys.path.append(str(ROOT)) # add ROOT to PATH
ROOT = Path(os.path.relpath(ROOT, Path.cwd())) # relative
# def detect(opt):
def detect():
webcam, source = True, "0"
# init
conf_thres = 0.45
iou_thres = 0.25
classes = None
agnostic_nms = False
max_det = 30
imgsz = [640, 640]
# Initialize
device = "cuda:0"
half = False
device = select_device(device)
half &= device.type != 'cpu' # half precision only supported on CUDA
# Directories
yolo_model = "yolov5m.pt"
deep_sort_model = "osnet_ibn_x1_0_MSMT17"
if type(yolo_model) is str: # single yolo model
exp_name = yolo_model.split(".")[0]
elif type(yolo_model) is list and len(yolo_model) == 1: # single models after --yolo_model
exp_name = yolo_model[0].split(".")[0]
else: # multiple models after --yolo_model
exp_name = "ensemble"
exp_name = exp_name + "_" + deep_sort_model.split('/')[-1].split('.')[0]
# Load model
model = DetectMultiBackend(yolo_model, device=device, dnn=False)
stride, names, pt = model.stride, model.names, model.pt
imgsz = check_img_size(imgsz, s=stride) # check image size
# Dataloader
if webcam:
# show_vid = check_imshow()
cudnn.benchmark = True # set True to speed up constant image size inference
dataset = LoadStreams(source, img_size=imgsz, stride=stride, auto=pt)
nr_sources = len(dataset)
else:
dataset = LoadImages(source, img_size=imgsz, stride=stride, auto=pt)
nr_sources = 1
# initialize deepsort
config_deepsort = "deep_sort/configs/deep_sort.yaml"
cfg = get_config()
cfg.merge_from_file(config_deepsort)
# Create as many trackers as there are video sources
deepsort_list = []
for i in range(nr_sources):
deepsort_list.append(
DeepSort(
deep_sort_model,
device,
max_dist=cfg.DEEPSORT.MAX_DIST,
max_iou_distance=cfg.DEEPSORT.MAX_IOU_DISTANCE,
max_age=cfg.DEEPSORT.MAX_AGE, n_init=cfg.DEEPSORT.N_INIT, nn_budget=cfg.DEEPSORT.NN_BUDGET,
)
)
outputs = [None] * nr_sources
# Get names and colors
names = model.module.names if hasattr(model, 'module') else model.names
# Run tracking
model.warmup(imgsz=(1 if pt else nr_sources, 3, *imgsz)) # warmup
dt, seen = [0.0, 0.0, 0.0, 0.0], 0
for frame_idx, (path, im, im0s, vid_cap, s) in enumerate(dataset):
t1 = time_sync()
im = torch.from_numpy(im).to(device)
im = im.half() if half else im.float() # uint8 to fp16/32
im /= 255.0 # 0 - 255 to 0.0 - 1.0
if len(im.shape) == 3:
im = im[None] # expand for batch dim
t2 = time_sync()
dt[0] += t2 - t1
# Inference
pred = model(im, augment=False, visualize=False)
t3 = time_sync()
dt[1] += t3 - t2
# Apply NMS
pred = non_max_suppression(pred, conf_thres, iou_thres, classes, agnostic_nms, max_det=max_det)
dt[2] += time_sync() - t3
# Process detections
for i, det in enumerate(pred): # detections per image
seen += 1
if webcam: # nr_sources >= 1
p, im0, _ = path[i], im0s[i].copy(), dataset.count
p = Path(p) # to Path
s += f'{i}: '
annotator = Annotator(im0, line_width=2, pil=not ascii)
if det is not None and len(det):
# Rescale boxes from img_size to im0 size
det[:, :4] = scale_boxes(im.shape[2:], det[:, :4], im0.shape).round()
# Print results
for c in det[:, -1].unique():
n = (det[:, -1] == c).sum() # detections per class
s += f"{n} {names[int(c)]}{'s' * (n > 1)}, " # add to string
xywhs = xyxy2xywh(det[:, 0:4])
confs = det[:, 4]
clss = det[:, 5]
# pass detections to deepsort
t4 = time_sync()
outputs[i] = deepsort_list[i].update(xywhs.cpu(), confs.cpu(), clss.cpu(), im0)
t5 = time_sync()
dt[3] += t5 - t4
# draw boxes for visualization
if len(outputs[i]) > 0:
for j, (output, conf) in enumerate(zip(outputs[i], confs)):
bboxes = output[0:4]
id = output[4]
cls = output[5]
c = int(cls) # integer class
label = f'{id} {names[c]} {conf:.2f}'
annotator.box_label(bboxes, label, color=colors(c, True))
LOGGER.info(f'{s}Done. YOLO:({t3 - t2:.3f}s), DeepSort:({t5 - t4:.3f}s)')
else:
deepsort_list[i].increment_ages()
LOGGER.info('No detections')
# Stream results
im0 = annotator.result()
if True:
cv2.imshow(str(p), im0)
cv2.waitKey(1)
# Print results
t = tuple(x / seen * 1E3 for x in dt) # speeds per image
LOGGER.info(f'Speed: %.1fms pre-process, %.1fms inference, %.1fms NMS, %.1fms deep sort update \
per image at shape {(1, 3, *imgsz)}' % t)
if __name__ == '__main__':
with torch.no_grad():
detect()
上記のコードは、yolov5-tracking が PC備え付けのカメラで動くコードとなります。
track_custom.py を実行すると、以下のような出力が得られます。
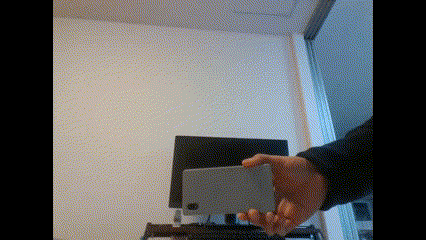
コードの説明
コードの説明をしていきます。ある程度は Yolov5 と被っているので、まずはこちらをご参考ください。
初期化
def detect():
webcam, source = True, "0"
# init
conf_thres = 0.45
iou_thres = 0.25
classes = None
agnostic_nms = False
max_det = 30
imgsz = [640, 640]
# Initialize
device = "cuda:0"
half = False
device = select_device(device)
half &= device.type != 'cpu' # half precision only supported on CUDA
ここら辺は yolov5 と同じです。
max_det=30 を調整すると、追跡する数が減るので、場面に応じて使用してください。
yolov5 のモデルを指定する
# Directories
yolo_model = "yolov5m.pt"
deep_sort_model = "osnet_ibn_x1_0_MSMT17"
if type(yolo_model) is str: # single yolo model
exp_name = yolo_model.split(".")[0]
elif type(yolo_model) is list and len(yolo_model) == 1: # single models after --yolo_model
exp_name = yolo_model[0].split(".")[0]
else: # multiple models after --yolo_model
exp_name = "ensemble"
exp_name = exp_name + "_" + deep_sort_model.split('/')[-1].split('.')[0]
yolo_model で使用する yolo のモデルを指定します。
deep_sort_model は、変更せずそのままで問題ありません。
どうしても使用したい場合は、こちらを参考に変更してください。
deep_sort の初期化
# initialize deepsort
config_deepsort = "deep_sort/configs/deep_sort.yaml"
cfg = get_config()
cfg.merge_from_file(config_deepsort)
先ほどの deep_sort_model と同様に、特別なことがない限り、config_deepsort を変更する必要がありません。
yolov5 の結果を取得し、画像に描画
# draw boxes for visualization
if len(outputs[i]) > 0:
for j, (output, conf) in enumerate(zip(outputs[i], confs)):
bboxes = output[0:4]
id = output[4]
cls = output[5]
c = int(cls) # integer class
label = f'{id} {names[c]} {conf:.2f}'
annotator.box_label(bboxes, label, color=colors(c, True))
LOGGER.info(f'{s}Done. YOLO:({t3 - t2:.3f}s), DeepSort:({t5 - t4:.3f}s)')
Yolo の推論結果から id, label, conf, bbox を取得し、annotator.box_label でim0 に描画します。
オリジナルの画像が欲しい場合は im0.copy() で別の画像を作成しておいてください。
ここで id は、物体追跡で使用しているIDです。特定の物体のみを追跡したい場合は id で管理してください。
推論結果を表示
# Stream results
im0 = annotator.result()
if True:
cv2.imshow(str(p), im0)
cv2.waitKey(1)
annotator から画像を取得し、表示します。
おわりに
今回は、Yolov5 で物体追跡を実行する Yolov5-tracking について説明しました。
一時期は追跡プログラムを自作していましたが、今回紹介した方の追跡プログラムは圧倒的に精度が良いです。
次回は、Yolov8-tracking の説明をしようと思います。
コメント